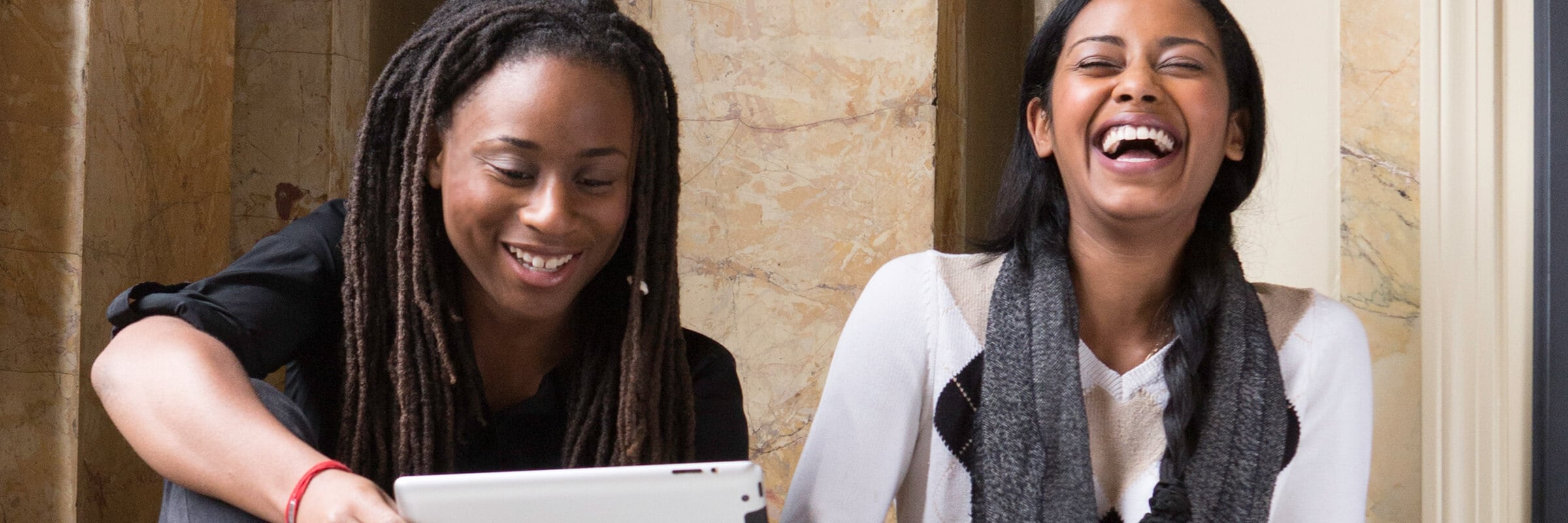
Pearson+
All in one place. Pearson+ offers instant access to eTextbooks, videos and study tools in one intuitive interface. Students choose how they learn best with enhanced search, audio and flashcards. The Pearson+ app lets them read where life takes them, no wi-fi needed. Students can access Pearson+ through a subscription or their MyLab or Mastering course.