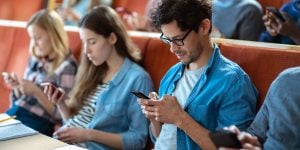
Voices of Innovation: A Q&A Series on Generative AI - Part 2
Using technology to improve teaching and learning is in Pearson’s DNA. As the first major higher education publisher to integrate generative AI study tools into its proprietary academic content, Pearson is excited to be harnessing the power of AI to drive transformative outcomes for learners. We are focused on creating tools that combine the power of AI with trusted Pearson content to provide students with a simplified study experience that delivers on-demand and personalized support whenever and wherever they need it.
In this multi-part blog series, you’ll have a chance to hear about AI innovations from Pearson team members, faculty, and students who have been involved in the development and rollout of Pearson’s AI-powered study tools.
In Part II, hear from Chris Hess, former professor and current Director of Higher Ed Product Managment for Physical Sciences, Math, and Statistics and Emily Ockay from Higher Ed Product Management with a focus on Pearson+ and eTextbooks. Chris and Emily are part of amazing teams at Pearson working diligently to bring the power of AI thoughtfully into Pearson learning platforms.


What has been your vision for bringing an AI-powered study tool into higher ed courseware and eTextbooks at Pearson?
Chris: We use the term “office hours” for a reason. When I was a professor, having a student come in who was struggling on a topic, and then helping diagnose and overcome that struggle and watch the light bulb go on…it was magic.
But most students don’t come to office hours, and the ones that did often already knew the answer and wanted validation, or they were struggling so badly that we often discussed alternate majors they might want to try. The 90% that never came to office hours still needed that kind of help -- at the exact moment they were struggling.
I started thinking about how to deliver a decent approximation of the office hours experience for those who couldn’t or wouldn’t go see a human expert.
My vision was to encapsulate the 'eureka' moments of office hours, extending that magic to every student at the precise time they needed it. The AI study tool is our way of democratizing access to those enlightening interactions, ensuring that no student is left in the dark due to scheduling conflicts or a hesitation to seek help.

Emily: I’ve worked in the higher ed market for over a decade, and one thing I’ve heard repeatedly from students is that they are overwhelmed by all the resources they’re juggling and the concepts they need to learn to get the grades they want.
When they study, they have 17 tabs open with lecture notes, the eTextbook, past homework, Google, YouTube, ChatGPT, etc., and it’s unclear which resources will be most helpful. I remember feeling overwhelmed by this when I was a student!
The other thing we know about students today is that they rarely sit down, crack open the eTextbook, and learn by reading linearly week after week. They use it as a reference to help them complete graded assignments and study for exams. And that’s okay! It’s just a different way of learning.
With the emergence of generative AI, our vision was to reimagine the eTextbook experience by infusing it with valuable study tools that we know students use and making it the trusted, go-to resource for students when they’re stuck or studying at 2:00 a.m.
What were some of your initial projections or goals set before launch? Has anything surprised you since bringing the AI study tool to market in MyLab, Mastering, and eTextbooks?
Chris: Really, just getting it out the door and live to customers felt like Sisyphus pushing a boulder up a hill at times!
Our herculean effort to launch was ambitious, targeting broad integration and substantial user engagement across the available content in our courseware. Yet, beyond numbers, my core aspiration was to witness our tool becoming a catalyst for learning—something that students and educators would not only use, but view as a transformative innovation in education.
Emily: Speed! One of our main goals was to deliver an AI-powered capability within the eTextbook thoughtfully and quickly, so we could learn more about how students use generative AI tools in an academic setting.
We also want to be able to make rapid improvements and smart decisions about what comes next. A mantra we used often during those early months of development was “maximize learning.”
Our other goals were to build something that students use repeatedly and that they find helpful in a real class setting, which (based on an in-product survey at the end of the semester) the majority did!
One interesting thing we learned was that there was a relationship between AI study tool usage and overall eTextbook engagement. Based on an analysis from our Efficacy & Learning team, AI tool users were over 3x more likely to remain or become what we would consider an efficient/active eTextbook user, rather than a passive user.
This is from just one semester of data so far, but it’s a trend that we’ll be watching closely.
How is Pearson's AI-powered study tool different - or aiming to be different - than other LLMs / AI tools like ChatGPT, Bard, etc.?
Chris: Pearson's AI distinguishes itself by its precision and pedagogical focus. Unlike general LLMs, our AI is trained on the answer, which ensures pinpoint accuracy. Instead of just spoon-feeding students what they need to get their points and move on, we focus on guiding them to understand how to get there.
It is also designed with a commitment to enduring knowledge transfer, laying the foundation for academic success beyond immediate assistance.
Emily: Pearson’s AI study tools are plugged into and informed by the assignments and content that faculty chose for the course. We assumed instructors would value that, but we didn’t anticipate how important that would be for students, too.
The students could easily ask ChatGPT or Gemini for a summary or for practice questions, but they choose to ask the eTextbook AI study tool instead because they know that the response will be more relevant to their instructor, assignments, and success in the course.
What improvements or optimizations have been made to the study tool based on user feedback or data gathered during the beta period? Can you provide examples of how customer insights have influenced development?
Chris: Iterative refinement has been key. We’ve evolved our chat strategy based on robust interaction data, enhancing our approach with more layered questioning and adaptive feedback that we call “nudges” that make students think, but doesn’t just give them the answer.
This evolution is a direct outcome of feedback available in the product (thumbs up/down), instructor focus groups, and student interviews. We still have a backlog of additional things we want to do based on what we learned in these sessions!

Emily: The Product, Engineering, and UX teams are constantly reviewing chatbot logs, in-product feedback, and user data to make iterative improvements.
One example is when we learned after the launch that some students found the AI study tool icon’s blinking animation behavior distracting. We reacted quickly to reduce blinking frequency and introduce a “sleep mode.”
We’ve also invested a lot in the chatbot’s conversation design to make it easier for students to get summaries, ask for explanations, and answer practice questions in a single, continued conversation. These longer exchanges are where deeper learning and “aha” moments happen.
Considering the rapidly evolving landscape of AI, how do you approach staying ahead of the curve in terms of innovation and what do you anticipate the next big trends for teaching and learning with AI will be?
Chris: It sometimes feels a bit like the Red Queen in “Alice in Wonderland” running as fast as she can just to stay in place, doesn’t it?!
Right now, I think we are helping students better than anyone at the moment on an individual problem. That is a dream come true for me, very much validation for my decision to leave the classroom. But what I really aspire to do is make a student’s overall learning path as efficient as it can possibly be.
I used AI software for a long time to train for triathlons. Every day I would get a workout that was designed to be exactly what I needed to improve the most, based on my current fitness and an understanding of past users who were like me.
Pearson has a mountain of data on learners, and we aspire to create similar experiences for users learning chemistry, biology, or mathematics.
It is hard to beat the feeling you get when you cross the finish line after a grueling swim, bike, and run, but watching students cross that stage for graduation because we provided something that made it possible comes pretty close.
Emily: The degree to which AI is now expected from almost every industry going forward will bring increased efficiency to all fields, including education. It will affect how Pearson and its authors create and maintain content, how instructors manage and teach their courses, and how students study and learn.
What I’m really excited about is what these innovations will free us up to do instead.
For example, I would love to see more time spent on developing, teaching, and studying authentic assessment. The deeper understanding that comes from real-world applications is invaluable as students think about their career goals beyond the classroom!