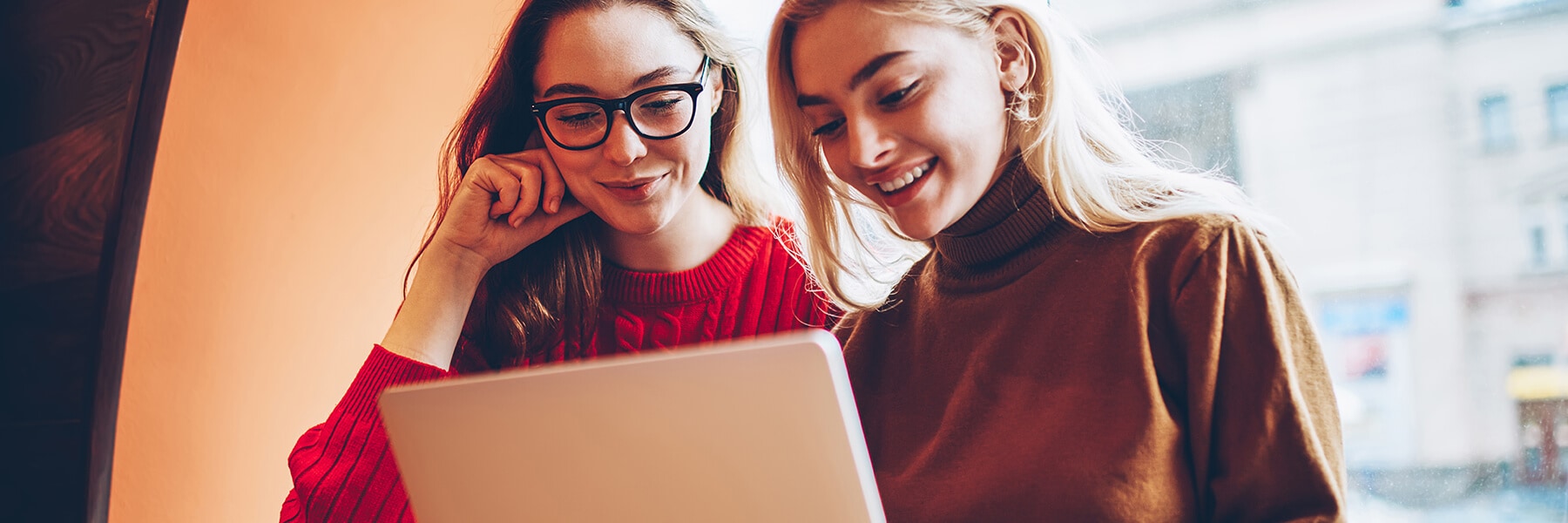
MyLab
Customize your course to teach your way. MyLab® is a flexible platform merging world-class content with dynamic study tools. It takes a personalized approach designed to ignite each student's unique potential. And, with the freedom it affords to adapt your pedagogy, you can reinforce select concepts and guide students to real results.